Unsupervised tree boosting for learning probability distributions
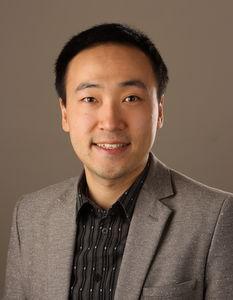
The DEMS Statistics Seminar series is proud to host
Li Ma
(Duke Univeristy)
Abstract
Based on additive ensembles of weak learners often in the form of regression and classification trees, boosting is among the most popular and powerful supervised learning algorithms, capable of achieving low bias and variance while overcoming the curse of dimensionality. Following the intuition that the additive tree model fitting approach also holds promise for addressing similar challenges in unsupervised settings, we consider the quintessential unsupervised problem of learning an unknown sampling distribution based on an i.i.d. sample, and propose an unsupervised tree boosting algorithm for inferring the underlying distribution. Integral to the algorithm is a new concept of “addition” of probability distributions that lead to a coherent notion of “residualization”, i.e., subtracting a probability distribution from an observation. We show that these notions arise naturally for univariate distributions in terms of cumulative distribution function (CDF) transforms and CDF compositions due to several “group-like” properties of univariate CDFs. In contrast, the traditional multivariate CDF does not preserve these properties and hence it does not lead to similar notions of “addition” and “residualization”. We show that a new definition of multivariate CDF can restore these “group-like” properties of the univariate CDF, thereby allowing the proper notions of “addition” and “residualization” to be formulated for multivariate distributions as well. This then gives rise to a simple boosting algorithm based on forward-stagewise fitting of an additive ensemble of tree-based models, which can be decision-theoretically justified in terms of sequential reduction of Kullback-Leibler divergence. The algorithm allows analytic evaluation of the density function for the fitted distribution and outputs a generative model with this density, which allows drawing Monte Carlo samples from the fitted distribution in a straightforward manner. Traditional wisdom in applying supervised boosting—such as setting the appropriate level of shrinkage/regularization—carry over into the unsupervised counterpart and leads to significant performance improvement. In particular, we introduce a new scale-dependent shrinkage strategy that utilizes a scale-specific learning rate. Moreover, we incorporate a two-stage fitting strategy that first fits the marginals followed by the copula of the distribution. We introduce a measure of variable importance based on the fitted tree ensemble, which gauges the relevance of each dimension in characterizing the underlying distribution. Finally, we show through benchmark datasets that our algorithm is competitive to the state-of-the-art deep learning-based normalizing flows in multivariate density estimation at a tiny fraction of their computational cost for training.
The seminar will be in person. Room: Aula del consiglio (U7 building – 4th floor)