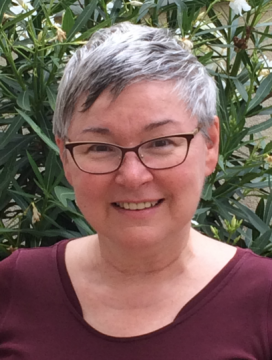
We are pleased to announce, for the series of DEMS Statistics Seminars, the seminar of Prof. Sylvia Frühwirth-Schnatter (Department of Finance, Accounting, and Statistics, WU Vienna University of Economics and Business, Vienna, Austria).
Time: Thursday, February 8th, at 12:00 pm
Place: University of Milano-Bicocca | DEMS | Room 2104 (building U7 Civitas, 2nd floor)
Title: Recent advanced in finite mixture analysis
Speaker: Sylvia Frühwirth-Schnatter (WU Vienna University of Economics and Business)
Abstract:
Since Karl Pearson's seminal work in 1894, finite mixture models have attracted a lot of attention due to their versatility in analyzing statistical data as well as due to the challenges in model estimation. Finite mixture models allow to infer latent heterogeneity in possibly large data sets and have a wide range of applications in economics and business, the social sciences or the life sciences, just to mention a few fields. The first part of this talk will provide a brief overview of the concept of finite mixture models and their application for model-based clustering and will discuss why it is particularly useful to approach finite mixture analysis from a Bayesian perspective.
The second part discusses recent advances in Bayesian mixture models when the number of components of the mixture distribution is unknown. Special emphasis will be placed on the framework of generalized mixtures of finite mixtures. It is shown that for this model class a pronounced difference between the number of components in the mixture distribution and the number of clusters in the data set exists. The concentration parameter in the Dirichlet prior on the mixture weights is instrumental in this respect and, for appropriate choices, finite mixture models imply a prior distribution on the partition of the data with a random number of data clusters. In addition, a prior is put on the number K of components in the mixture distribution. This allows to infer simultaneously K and the number of data clusters from the data.
Finite mixtures are often considered to be quite different from infinite mixtures, commonly used in Bayesian non-parametric mixture analysis. By characterizing the induced partitions in a unifying framework, it is shown that dynamic mixtures of finite mixtures where the hyperparameter of the Dirichlet prior decreases as the number of components K increases are a natural extension of Dirichlet process mixtures beyond Gibbs-type priors and discuss their relationship to mixtures of Pitman-Yor-Process mixtures.
Finally, a novel MCMC algorithm for posterior inference in finite mixtures, the telescoping sampler, will be discussed. Most importantly, the telescoping sampler avoids the tedious design of moves in alternative trans-dimensional approaches such as reversible jump MCMC. The telescoping sampler is straightforward to apply even to high-dimensional data sets and arbitrary component models, as will be illustrated by various applications, including multivariate mixtures of Gaussians, and mixtures of factor analyzers.
(based on joint work with Bettina Grün, Gertraud Malsiner-Walli, and Margaritha Grushanina)