Deep learning to play games
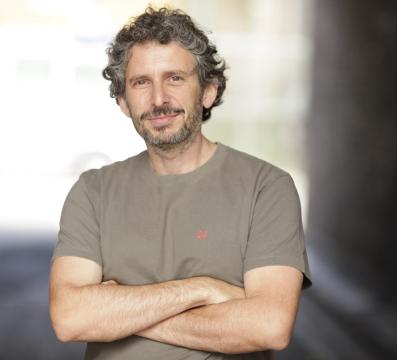
The DEMS Economics Seminar series is proud to host
Daniele Condorelli
(University of Warwick)
with M. Furlan
ABSTRACT
We train two neural networks adversarially to play normal-form games. At each iteration, a row and column network take a new randomly generated game and output individual mixed strategies. The parameters of each network are independently updated via stochastic gradient descent to minimize expected regret given the opponent’s strategy. Our simulations demonstrate that the joint behavior of the networks converges to strategies close to Nash equilibria in almost all games. For all 2 × 2 and in 80% of 3 × 3 games with multiple equilibria, the networks select the risk-dominant equilibrium. Our results show how Nash equilibrium emerges from learning across heterogeneous games.
The seminar will be in presence, Seminar Room 2104, Building U7-2nd floor