Stratified stochastic variational inference for networks
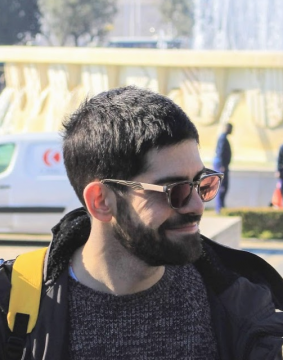
Emanuele Aliverti
(University of Padua)
Abstract: There has been considerable interest in the Bayesian modelling of high dimensional networks via latent space approaches. These methods allow one to characterize the dependence structure of a network with simplicity, often demonstrating remarkable empirical performances. Unfortunately, as the number of nodes increases, estimation based on Markov chain Monte Carlo becomes very slow and can lead to inadequate mixing. In this talk, I will illustrate scalable algorithms to conduct approximate posterior inference for latent space models, relying on a novel stratified variational inference approach that leverages the sparsity of the network structure. The benefits of the proposed methods will be illustrated with different case studies, focusing on high-resolution brain imaging and investigating the relationships among contrade in Venice during the 18th century.