Model selection by sparse composition of estimating equations
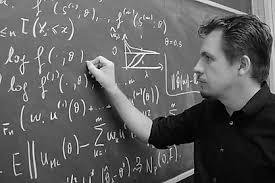
As part of the DEMS Statistics Webinar series Professor Davide Ferrari (University of Bolzano) will present the paper:
“Model selection by sparse composition of estimating equations”
Wednesday, December 16, 13:00
Abstract:
This talk introduces a method for selecting high-dimensional models based on a truncation mechanism to generate sparse estimating equations. Given a set of low-dimensional estimating equations for the model parameters, a high-dimensional model is selected by minimizing the distance between a composite estimating equation and the full likelihood scores subject to a L1 -type penalty. The proposed strategy reduces the overall model complexity scores subject to a L1 -type penalty. The proposed strategy reduces the overall model complexity by dropping the noisy terms in the estimating equations. Differently from other approaches to model selection, our penalty involves the inclusion of low-dimensional equations rather than model parameters; this implies that consistency of the final parameter estimates remains largely unaffected by the selection mechanism. The numerical and statistical efficiency final parameter estimates remain largely unaffected by the selection mechanism. The numerical and statistical efficiency of the new methodology is illustrated through examples on simulated and real data.