PRODUCT vs MIXTURE OF EXPERTS: ON DISTRIBUTED GAUSSIAN PROCESS REGRESSION
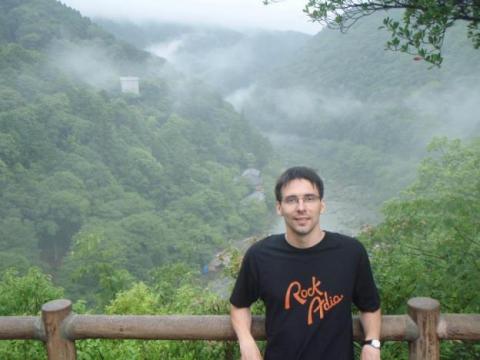
The DEMS Statistics Seminar series is proud to host
Botond Szabo
(Bocconi University)
Abstract:
Gaussian Process (GP) are arguably one of the most popular choices of priors in Bayesian nonparametrics, however, they typically scale poorly with the data size n even in the standard nonparametric regression model, i.e. the computational complexity of training and predicting are O(n3) and O(n2), respectively. This considerably limits their practical applicability for big data sets. Various methods have been proposed to speed up the computations. In this talk we focus on distributed Bayesian techniques, where the data is divided over local machines/cores and the posteriors are computed locally in parallel to each other based on the partial data sets. Then in the final step the local posteriors are aggregated into a global approx- imation of the original posterior distribution. We derive theoretical guarantees and limitations for these procedures in context of the nonparametric regression model.
Depending on how the data are divided over the local machines we distinguish two main classes of approaches, the product and mixture of GP experts. In the product of experts the data are divided randomly, each local machine receiving ob- servations over the whole domain of the regression function. In the mixture of experts the data are divided spatially, each local machine receiving all the observations corresponding to a given small region or bin. We show that although several of the proposed approaches provide bad estimation and unreliable uncertainty quantifica- tion, appropriately tuned methods can achieve the minimax contraction rate and provide asymptotically valid confidence sets in case we know the regularity of the true regression function of interest. However, if the regularity is unknown, the state- of-the-art distributed data driven procedures provide sub-optimal contraction rates, failing to adapt to the underlying functional classes. In contrast to this we prove that mixture of experts methods provide optimal contraction rates both in the adaptive and non-adaptive setting. We also propose a new averaging method for the local posteriors, which deals with the discontinuity problem arising in mixtures of experts. (with Amine Hadji, Harry van Zanten, and Aad van der Vaart)
References
[1] Amine Hadji and Botond Szabo Optimal recovery and uncertainty quantification using distributed Gaussian process regression. Working paper.
[2] Amine Hadji, Botond Szabo and Aad van der Vaart Adaptation with mixture of Gaussian Process experts. Working paper.
[3] Botond Szabo and Harry van Zanten An asymptotic analysis of distributed non- parametric methods., Journal of Machine Learning Research 20 (2019) 1-30.
DEMS seminar room, Building U7 Civitas, 2nd floor.